Reduce your inventory costs and truck rolls with accurate parts prediction
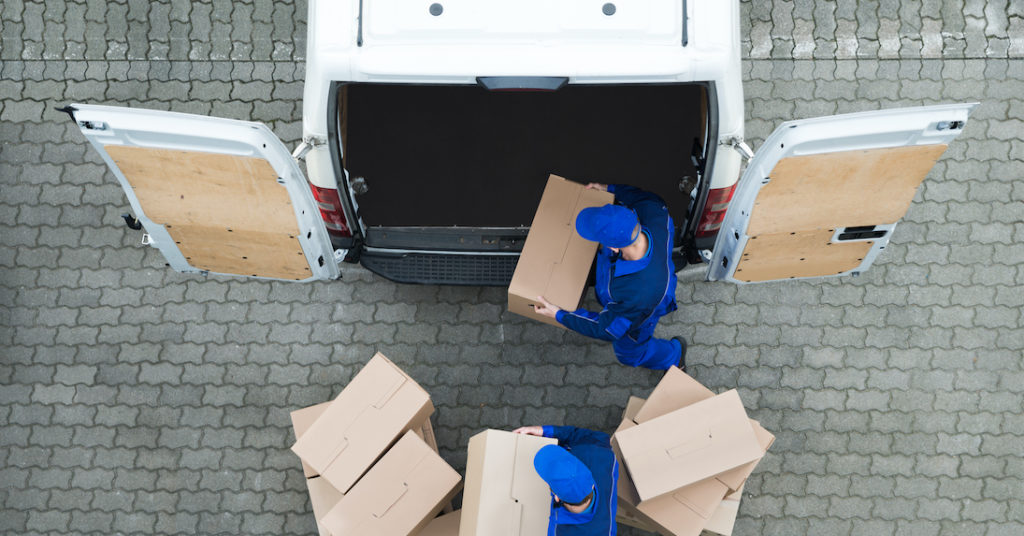
The latest machine-learning tech can determine which parts, and how many, to carry in the warehouse and on the service truck
By Andy Chinmulgund
The COVID-19 pandemic is redefining business practices across industry verticals. As social distancing and shelter in place directives persist, the service industry is reinventing onsite service visits. Any onsite visit is a safety concern for both the technician and the consumer, so minimizing these is a priority, which means showing up with the right part and fixing a technical problem in the first visit.
Predicting the right parts for any given job comes down to experience. From handling simple repairs of residential appliances to complex issues with medical equipment, service contractors and field technicians often rely on years of field-service experience when stocking their trucks with right mix spare parts. In the absence of hard data to guide them, they will err toward caution and carry frequently used parts but not necessarily the ‘right’ parts. This guesswork can mean multiple service visits, which can cost the manufacturer service companies an additional $150 to $500.
It starts when the ticket is opened
When consumers reach out to a contact center to report a problem, agents typically record the problem as described and hand off to the service team to determine the problem. So, for example, if the customer states that there’s “water under the fridge and maybe the ice maker is leaking,” the agent records the problem and the symptoms without really understanding the root cause. This sets in motion a series of decisions and actions that may not fix the problem.
What if the contact center agent had access to an intelligent assistant that can prompt the right set of questions to accurately determine the root cause? This assistant, which applies machine learning to understand hundreds of previous service tickets for water leaks with this model of fridge, could direct the agent to ask key questions that finally pin-point the problem: the inlet water valve.
So now, instead of dispatching a service tech with an expensive ice-maker assembly, we will roll a truck with a simple valve assembly. And, we will fix it right the first time, which reduces costs, addresses safety concerns, and makes the customer happy.
Bruviti technology in the loop
Bruviti’s parts prediction model is trained on service history records and warranty claims data supplied by equipment manufacturers. The warranty data is matched with a corresponding consumer problem description data. Then, using natural-language processing, problem descriptions are extracted from the service ticket data, while used parts data is extracted from actual warranty claims. Specific part numbers are mapped to the corresponding parts description data and the model is fine-tuned as per appliance models. The model is retrained at regular intervals by linking future claims and ticket data.
Evaluate the model performance
The recall rate is a good measure to evaluate model performance. Let’s take for example the model predicts parts a, b, c, d and the servicer’s warranty claim data shows actual parts used to be a, b, c, d, e. The recall rate is calculated at 80%.
Bruviti’s parts prediction model performance improves over time with continuous re-training as additional data gets fed into the model.